Harnessing Machine Learning for Control Systems in Business
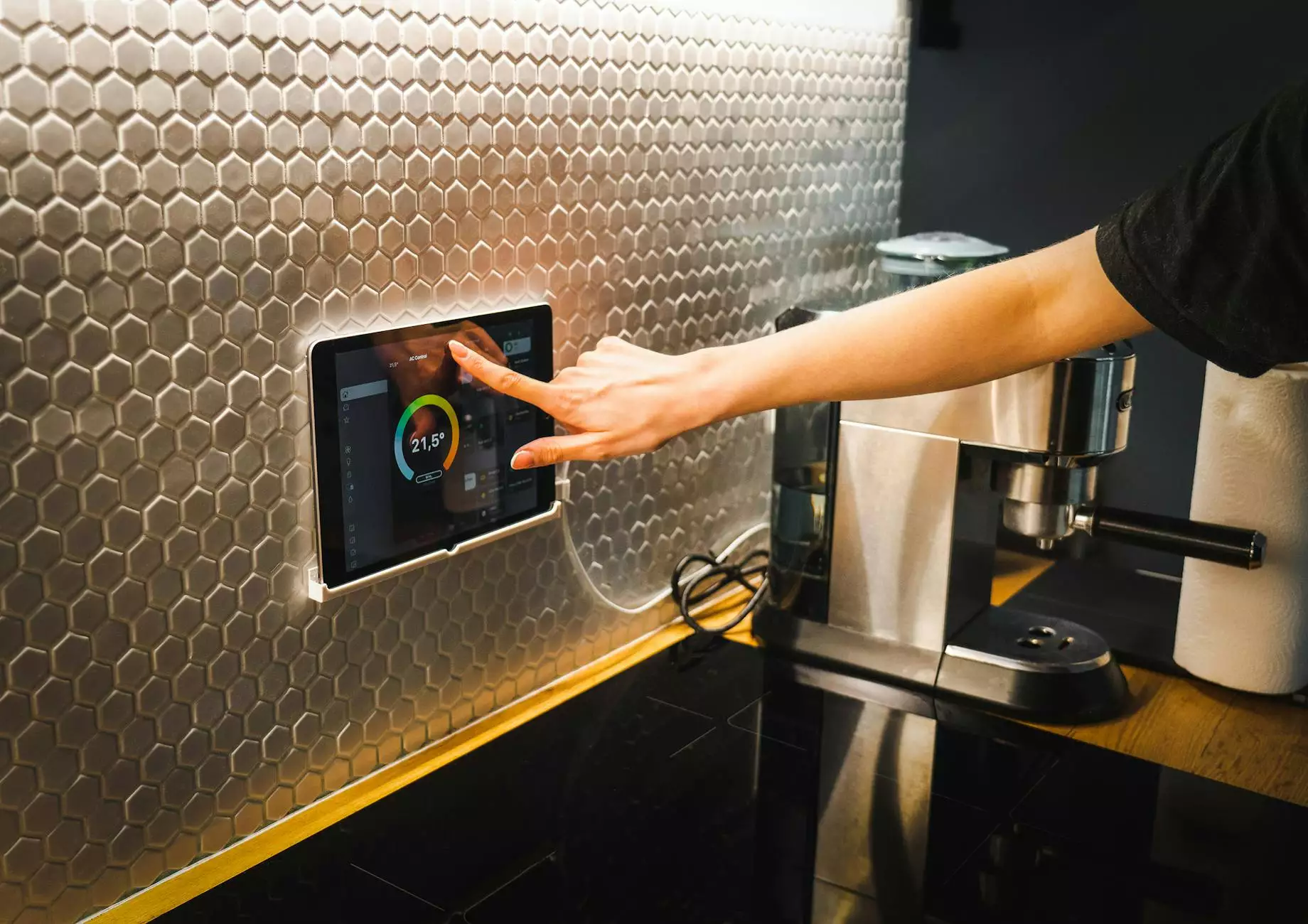
In today's fast-paced business environment, innovation is not merely an option but a necessity. Companies striving to maintain competitive edges are turning towards advanced technologies, amongst which, machine learning for control systems is emerging as a formidable tool. This technology promises unprecedented efficiency and accuracy in business operations, enabling organizations to streamline processes and make data-driven decisions.
The Basics of Machine Learning in Control Systems
Machine learning is a subset of artificial intelligence that empowers systems to automatically learn and improve from experience without explicitly being programmed. In the context of control systems, machine learning algorithms analyze data patterns, enabling businesses to fine-tune their operations accordingly. This adaptability is crucial for managing dynamic business environments.
Understanding Control Systems
A control system consists of devices or groups of devices that manage, command, direct, or regulate the behavior of other devices or systems. It plays a vital role in various industries including manufacturing, transport, and energy. By integrating machine learning, control systems can enhance their efficiency through predictive analytics and real-time adjustments.
Key Benefits of Implementing Machine Learning for Control Systems
- Increased Efficiency: Machine learning algorithms can optimize processes by analyzing historical data and predicting future performance, leading to significant improvements in productivity.
- Intelligent Automation: Businesses can automate routine tasks by employing intelligent systems that learn continuously and adapt to new information, minimizing human error.
- Enhanced Decision Making: With predictive analytics, machine learning for control systems allows businesses to make informed decisions based on actionable insights derived from comprehensive data analysis.
- Cost Reduction: Efficient resource management through machine learning helps in reducing operational costs, significantly impacting the bottom line.
- Improved Quality: By configuring control systems that learn from operational data, businesses can sustain high quality and consistent products or services.
Applications in Business
The applications of machine learning for control systems extend across multiple business sectors. Here are a few notable implementations:
1. Manufacturing Automation
In manufacturing, machine learning algorithms can analyze sensor data from machinery to predict failures before they occur, facilitating proactive maintenance. This approach results in less downtime and reduced maintenance costs.
2. Supply Chain Optimization
Machine learning models can be employed to forecast demand accurately, enabling better inventory management and cost-effective logistics solutions. By anticipating shifts in consumer behavior, businesses can adjust their supply chain strategies dynamically.
3. Energy Management
In energy sectors, companies utilize machine learning for real-time monitoring and dynamic adjustment to optimize energy consumption and reduce wastage. Smart grids, powered by machine learning, are revolutionizing how energy is distributed and consumed.
Integrating Machine Learning into Your Business Strategy
To effectively harness the power of machine learning for control systems, businesses must devise a comprehensive strategy that encompasses the following steps:
Step 1: Data Collection
Data is the lifeblood of machine learning. Collecting relevant and high-quality data from operations is essential. This can be achieved using IoT devices and advanced sensors that provide real-time data streams.
Step 2: Data Governance
Implementing a robust data governance system is critical. Businesses need to ensure that data used for machine learning complies with policies and regulations, guaranteeing data privacy and security while also maintaining data integrity.
Step 3: Model Development
After data collection and governance, the next step is to develop and train machine learning models. This involves selecting appropriate algorithms that align with business objectives and utilizing tools like TensorFlow or PyTorch for model training.
Step 4: Continuous Learning and Adaptation
Post-implementation, systems should be equipped to learn and adapt continuously. Utilizing a business process automation service, organizations can automate updates and refine models as new data is acquired, ensuring sustained relevance and accuracy of predictions.
Challenges in Implementing Machine Learning for Control Systems
Though promising, the integration of machine learning into control systems is not without challenges:
- Data Quality: Poor data quality can adversely affect model accuracy, highlighting the necessity for stringent data validation processes.
- Skill Gap: Organizations may face a shortage of skilled data scientists who can develop and implement machine learning models effectively.
- Change Management: Integrating machine learning into existing systems may require substantial changes to workflows and processes, necessitating comprehensive training and education for staff.
- Cost Considerations: The initial investment in technology and talent can be substantial, though long-term benefits often outweigh upfront costs.
The Future of Machine Learning in Business
The potential of machine learning for control systems is vast and continues to grow. As technologies become more sophisticated, businesses can expect:
1. Advanced Predictive Analytics
The evolution of machine learning will foster more advanced predictive analytics, enhancing the ability of businesses to anticipate market trends and customer needs.
2. Greater Personalization
Companies will be able to deliver more personalized products and services by analyzing customer data more effectively, creating tailored experiences that enhance customer satisfaction and loyalty.
3. Sustainable Practices
With the increasing focus on sustainability, machine learning can play a crucial role by optimizing resource usage and minimizing waste in manufacturing and energy sectors.
Conclusion
In summary, harnessing machine learning for control systems is not just a trend but a fundamental shift in how businesses operate and progress in their respective fields. Organizations that adopt this technology will likely experience increased efficiency, smarter automation, and improved decision-making capabilities that pave the way to future success. At Intalio.com, we specialize in delivering robust solutions that incorporate machine learning, data governance, and automation services tailored to meet the unique needs of today's businesses.
Get Started with Machine Learning Today
If you're ready to take your business to the next level by leveraging the power of machine learning for control systems, contact us today at Intalio.com. Together, we can unlock new potentials and drive your business toward unmatched operational excellence.